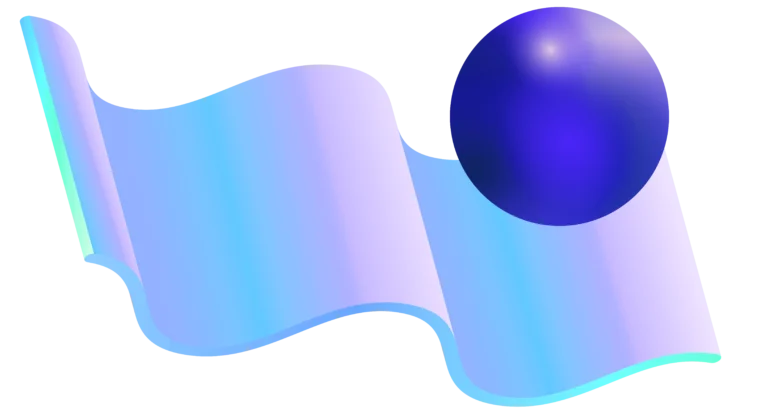
Datasets
We provide high-quality, curated datasets to accelerate AI model development, ensuring accuracy, accessibility, and ethical data practices for impactful research.
Funder: Lacuna
This dataset comprises images of five crop classes in the training set: cassava, sugarcane, maize, cashew, and coffee. It is the first part of a larger crop classification dataset, with the second part containing additional classes (weeds and unknown images), along with validation and test data.
When combined, the full dataset is structured into three splits: train, validation, and test, with data augmentation applied to the training set. In total, there are 4,074 images across all seven classes, with 582 images per class. Data collection involved multiple methods, including video recordings from in-field gardens, image extraction, and high-resolution drone imagery. The dataset was developed in collaboration with Makerere AI Lab, Uganda Marconi Lab, the National Coffee Research Institute, and the National Crops Resources Research Institute.
Funder:
This is the second part of the data. It consists of two classes that remained from the training set’s data: weeds and unknown. Plus, the validation and test data with all classes. Please, to use it, combine the first part with all training classes with this data.
Funder: Lacuna
This beans dataset was created to provide an open and accessible, well-labeled, sufficiently curated image dataset. This is to enable researchers to build various machine learning experiments to aid innovations that may include; bean crop disease diagnosis and spatial analysis. This beans image dataset was collected across three different classes: Healthy, Angular Leaf Spot (ALS), and Bean Rust.
Funder:
We present an image dataset of cassava root cross-sections collected from field trials alongside agricultural experts. The data set contains healthy cassava root images and images of cassava roots affected by Cassava Brown Streak Disease (CBSD). The data was collected from the National Crop Resources Research Institute (NaCRRI) and the Tanzania Agricultural Research Institute (TARI) that hosts the national cassava breeding programs of Uganda and Tanzania respectively. The dataset contains both clean and necrotized cassava roots. This raw dataset is publicly available as a Mendeley repository
Funder: Lacuna
We present a spectral dataset, procedures and steps we adopted to collect disease data in a controlled environment aiming at early disease detection in cassava. As a baseline, we extended these procedures to an open-field experiment. We collected visible and near-infrared spectra captured from leaves infected with two common cassava diseases. Together we collected plant image data from leaves where spectral data was captured. In this experiment, biochemical data was collected and taken as the ground truth. Finally, agricultural experts provided a disease score for each plant where data was collected. The process of disease monitoring and data collection took 19 and 15 consecutive weeks for screen house and open field respectively until disease symptoms were visibly seen by the human eye.
Funder: Lacuna
We present a spectral dataset that was collected from healthy and infected plants in a controlled environment (screenhouse) and in a field setup. The screen house setup rules out the influence of other diseases, pests or severe weather conditions while in an open field, crops grow under a natural environment, also exposed to crop pests. The experiment was carried out in partnership with the National Crop Resources Research Institute (NaCRRI). The dataset is composed of two experiments: screenhouse and open field experiment.
Funder: Lacuna
The dataset was created to provide an open-source and well-curated image dataset showing diseased and healthy cassava leaf images from Uganda. This will be used by data scientists, researchers, the wider machine learning community, and experts from other domains to conduct research into automating the identification and diagnosis of cassava crop diseases. The image dataset was collected across three different classes: Healthy, Cassava Brown Streak Disease (CBSD), and Cassava Mosaic Disease (CMD).
Funder: Lacuna
The dataset was created to provide an open and accessible maize dataset with well-labeled, sufficiently curated, and prepared maize crop imagery that will be used by data scientists, researchers, the wider machine learning community, and social entrepreneurs within Sub-saharan Africa and worldwide for various machine learning experiments so as to build solutions towards infield maize crop disease diagnosis and spatial analysis. The image dataset was collected across three different classes: Healthy, Maize Streak Virus (MSV), and Maize Leaf Blight (MLB).
Funder:
NONE YET
None Yet
Funder:
We present a dataset that contains images of whitefly-infested cassava leaves captured from cassava fields located at the National Crop Resources Research Institute (NaCRRI). The data contains images of adult whiteflies, which are one of the leading contributors to the spread of Cassava Brown Streak Disease (CBSD). The images were captured from the top open cassava leaves of randomly selected cassava plants. The technique used to capture the image data is suitable for monitoring the populations of adult whiteflies in the field,
Funder: Google
The dataset consists of sentences in the Luganda language that solely pertain to the agricultural domain. These sentences cover a wide range of topics within agriculture, such as farming, animal breeding, crop cultivation, crop storage and yield, marketing of produce, and environmental aspects. The dataset was created to provide a high-quality agriculture domain-specific dataset for the Luganda language that can be used in different use cases, including; Machine translation for agriculture, Language modelling, Topic modelling for agriculture, and Named Entity Recognition for agriculture.
Funder:
The datasets presented in this work consist of high-resolution images of coffee and cashew plants acquired using Unmanned Aerial Equipment (UAV) equipment from small and large-scale farms across Uganda. Images range approximately between 10 MB and 12 MB in size, approx. 4000 by 3200 pixels in dimension and 72 pixels/in in Dots per inch (DPI). Each image is annotated with multiple bounding boxes, each enclosing an object of interest. Each image is accompanied by metadata, including the date (timestamp) and the geographic location (latitude and longitude) where it was captured.
Funder: Lacuna
Acholi is a very low-resourced language spoken in parts of Northern Uganda. This dataset contains 40,037 Acoli sentences. The sentences were collected and evaluated by Acoli linguists with the collaboration of teams at Marconi Research and Innovation Lab and Makerere AI Lab from Makerere University.
Funder: Lacuna
This dataset contains a total of 39,999 sentences. The sentences are split into two separate files. One file contains 20,764 sentences from the Northern dialect and another one contains 19,235 sentences from the Southern dialect. This dataset was compiled by a team of Linguists and researchers from the Makerere AI and Data Science Research Lab and Marconi Research and Innovation Lab at Makerere University. This dataset was created with support from Lacuna Fund.
Funder: Lacuna
This dataset contains 100,000 Kiswahili sentences. We want to thank the team at the Makerere AI and Marconi Labs at Makerere University, TAVODET Youth Development (TYD) Innovation Incubator, Ai Kenya, Maseno University, United States International University-Africa (USIU-Africa), and Kabarak University who have worked tirelessly and collaboratively to source, create and prepare this Kiswahili monolingual dataset. This dataset was created with support from Lacuna Fund
This English-Luganda parallel sentence corpus consists of gendered examples created by a team of researchers from Makerere AI Lab at Makerere University with a team of Luganda teachers, students and freelancers. The collaborative work which involves generating English sentences under CC-0 and translating these sentences using a crowdsourcing, iterative and opensource approach was done using Pontoon an opensource Translation Management System built by Mozilla. This is a corpus of 1,000 parallel sentences.
Funder: Lacuna
This dataset contains 100,000 Luganda sentences. Luganda is a Bantu language and is one of the major languages spoken in Uganda. This dataset was compiled by researchers at the Makerere AI and Data Science Research Lab and Marconi Research and Innovation Lab. We want to thank the Department of African Languages, Makerere University and the Ekibiina Ky’Olulimi Oluganda (EKO) for the work done in curating the dataset.
Funder: Lacuna
This dataset contains 10,000 parallel sentiment-tagged sentences. English sentences were translated to both Luganda and Swahili. The translations were done by language experts and professional translators in collaboration with researchers at Makerere University. All sentences were tagged with a sentiment code. The sentiment tags were applied with respect to the English sentences.
Funder:
This is a partial multilingual parallel corpora of 5 East African languages. The dataset contains an English text corpus that has been translated into five East African languages: Acholi, Runyankore, Luganda, Lumasaba, and Swahili.
Funder: Lacuna
This dataset contains thick and thin blood smear images captured using smartphones on a microscope. The images have been annotated with bounding boxes showing different objects of interest in each image.